Making impossible measurements of cell and tissue dynamics
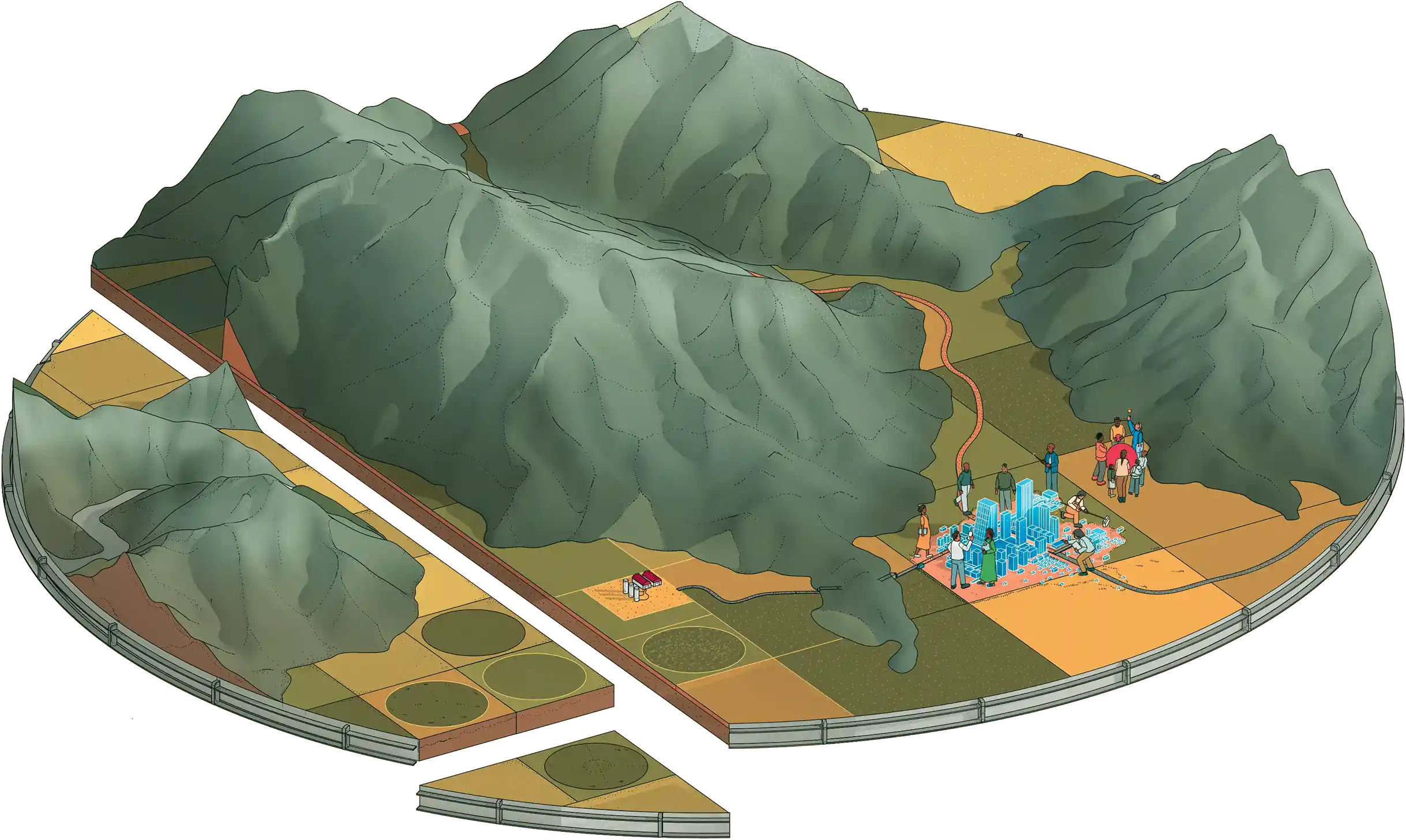
Challenge: The completion of the Human Genome Project two decades ago was the catalyst for a series of scientific and technological revolutions that have transformed our understanding of biology. The advances in next-generation DNA sequencing have dropped the cost and time taken for sequencing a human genome from the Human Genome Project’s >$1,000,000,000 in a decade to <$1,000 in under 24 hours. Single-cell genomics has given us a new window into the structure and function of biological systems, while genome editors like CRISPR allow us to modify them precisely and systematically. A new wave of spatially-aware genomics technologies and high-throughput microscopy promise to scale these insights from individual cells dissociated from their context to entire tissues and organs, and organoid platforms are enabling us to bring this growing technological arsenal to bear directly on human tissue.
Meanwhile, many of the biggest questions in biology and medicine today are about changes over time: How does a healthy cell develop into a malignant tumor? How does the immune system mobilize to generate an overwhelming antibody response adapted to a novel pathogen? How do our cells change as we age, and can these changes be slowed or reversed? Yet all of the new technologies above are inherently static and irreversible in nature: they can only be applied once to any particular sample at a single point in time, usually destroying it in the process, so many experiments interrogating changes over time are impossible to perform directly. Overcoming this limitation is thus a fundamental statistical and computational problem in modern biology whose solution will also require major advances in AI and machine reasoning.
Project: We aim to learn dynamical models of cell or tissue multi-omic state by integrating many kinds of incomplete causal knowledge, such as known mechanistic signals of temporal change, high-quality reference datasets, and experimental perturbations. We can use the models to partially identify outcomes of impossible experiments (like taking snapshots of the same sample twice).
Basis leads: Eli Bingham, Martin Jankowiak